class: middle .center[
# Bayesian Methods for Machine Learning .small-vspace[ ] ### Lecture 2 - Fundamentals of machine learning
.bold[Simon Leglaive]
.tiny[CentraleSupélec] ] .credit[This presentation is adapted from [INFO8010 - Deep Learning](https://github.com/glouppe/info8010-deep-learning) course by [Gilles Louppe](https://glouppe.github.io/) at ULiège.] --- class: middle ## In the last episode... We want to infer some latent information from observations. .alert-g-left[ - **Data**: Get .bold[unlabeled] data $\mathcal{D} = \left\\{\mathbf{x}\_i \overset{i.i.d}{\sim} p^\star(\mathbf{x})\right\\}\_{i=1}^N$. - **Modeling**: Define a model that relates the latent variable of interest to the observations $p(\mathbf{x},z; \theta) = p(\mathbf{x} \mid z; \theta) p(z; \theta)$. - **Inference**: Compute the posterior distribution $p(z \mid \mathbf{x} ; \theta)$, which can then be used in many different ways. - **Learning**: Estimate the unknown model parameters $\theta$ by maximizing the log-marginal likelihood $\ln p(\mathbf{x}; \theta)$ averaged over the dataset. ] .small-vspace[ ] This corresponds to a form of **unsupervised learning**, using a **generative** Bayesian modeling approach. --- class: middle ## Today We will focus on **supervised learning** with **discriminative** models. The key concepts you should be familiar with at the end of this course are the following: - Supervised learning - Generative vs. discriminative model - Empirical risk minimization - Multinomial logistic regression (lab session) --- class: middle, center # Supervised learning --- class: middle .alert-g[ The term **supervised** means that, **at training time**, the input data samples $\mathbf{x}\_i \in \mathcal{X}$ are **labeled** with the information we will try to infer **at test time**. The **labels** are represented by $y_i \in \mathcal{Y}$. ] .center.width-70[] .credit[Image credits: [Antoine Deleforge](https://members.loria.fr/ADeleforge/lectures/), Inria, course given at Télécom Physique Strasbourg.] --- class: middle ## Training time At **training time**, our observations consist of (input, label) pairs, which are assumed to be i.i.d according to some distribution $p^\star(\mathbf{x},y)$: $$\mathcal{D} = \Big\\{(\mathbf{x}\_i,y\_i) \in \mathcal{X} \times \mathcal{Y} \overset{i.i.d}{\sim} p^\star(\mathbf{x},y) \Big\\}\_{i=1}^N.$$ For instance, $\mathbf{x}\_i$ is a $D$-dimensional input feature vector and $y\_i$ is a scalar label (e.g., a category or a real value). --- class: middle ## Test time At **test time**, we only observe a new input data sample $\mathbf{x}$, from which we want to infer $y$. This means computing the **posterior** distribution $p(y \mid \mathbf{x} ; \theta)$, which depends on a set of parameters $\theta$ that have been estimated during the **learning** stage, at training time. In supervised learning, we are often only interested in a **point estimate** $\hat{y}$, for instance: - classification task: $$ \hat{y} = \underset{k \in \\{1,...,C\\}}{\arg\max}\, p(y=k \mid \mathbf{x} ; \theta ) \in \\{1,...,C\\}; $$ - regression task: $$ \hat{y} = \mathbb{E}\_{p(y \mid \mathbf{x} ; \theta )}\left[ y \right] \in \mathbb{R}. $$ --- class: middle ## Supervised learning with **generative** modeling We have access to a .bold[labeled] dataset $\mathcal{D} = \left\\{(\mathbf{x}\_i,y\_i) \overset{i.i.d}{\sim} p^\star(\mathbf{x},y) \right\\}\_{i=1}^N$. - **Generative modeling** - we define the joint distribution to explain how the input data $\mathbf{x}$ are generated from the label $y$: .small-nvspace[ ] $$p(\mathbf{x}, y; \theta) = p(\mathbf{x} \mid y; \theta) p(y; \theta).$$ - **Inference** - we "invert" this generative model using Bayes theorem: $$p(y \mid \mathbf{x} ; \theta) = \frac{p(\mathbf{x} \mid y; \theta) p(y; \theta)}{p(\mathbf{x}; \theta)}. $$ - **Learning** - we estimate the unknown model parameters $\theta$ by maximizing the log-likelihood $\ln p(\mathbf{x}, y; \theta)$ averaged over the dataset $\mathcal{D}$: $$\underset{\theta}{\max}\hspace{.1cm} \mathbb{E}\_{p^\star(\mathbf{x},y)} [ \ln p(\mathbf{x}, y; \theta) ] .$$ **No need to marginalize over $y$ as it is observed in a supervised setting!** --- ## Supervised learning with **discriminative** modeling We have access to a .bold[labeled] dataset $\mathcal{D} = \left\\{(\mathbf{x}\_i,y\_i) \overset{i.i.d}{\sim} p^\star(\mathbf{x},y) \right\\}\_{i=1}^N$. - **Discriminative modeling** - we define the joint distribution to directly explain how the label $y$ is obtained from the input data $\mathbf{x}$: $$p(\mathbf{x}, y; \theta) = p(y \mid \mathbf{x}; \theta) p(\mathbf{x}),$$ where $p(\mathbf{x})$ is non-informative (e.g., uniform after normalization) and **does not depend on** $\theta$. - **Inference** - super easy, just evaluate the model! - **Learning** - we estimate the unknown model parameters $\theta$ by maximizing the log-likelihood $\ln p(\mathbf{x}, y; \theta) = \ln p(y \mid \mathbf{x}; \theta) + cst$ averaged over the dataset $\mathcal{D}$: $$\underset{\theta}{\max}\hspace{.1cm} \mathbb{E}\_{p^\star(\mathbf{x},y)} \left[ \ln p(\mathbf{x}, y; \theta) \right] \hspace{.2cm}\Leftrightarrow\hspace{.2cm} \underset{\theta}{\min}\hspace{.1cm} \mathbb{E}\_{p^\star(\mathbf{x},y)} \left[ - \ln p(y \mid \mathbf{x}; \theta) \right] .$$ In supervised discriminative learning, $- \ln p(y \mid \mathbf{x}; \theta)$ is often called the **negative log-likelihood (NLL) function**, which can be confusing (e.g., [torch.nn.NLLLoss](https://pytorch.org/docs/stable/generated/torch.nn.NLLLoss.html)). --- ## Wrap-up .alert-g-left[ - **Data**: Get the labeled training dataset $\mathcal{D} = \left\\{(\mathbf{x}\_i,y\_i) \overset{i.i.d}{\sim} p^\star(\mathbf{x},y) \right\\}\_{i=1}^N$. - **Modeling/inference**: Define $p(y \mid \mathbf{x}; \theta)$. - **Learning**: Estimate $\theta$ by minimizing $- \ln p(y \mid \mathbf{x}; \theta)$ averaged over $\mathcal{D}$. ] - 3 fundamental examples are available in the appendix. We will study the multinomial classification example in detail during the lab session. -- - Having to define a model as the expression of a probability mass/density function $p(y \mid \mathbf{x}; \theta)$ can be restrictive, and is actually not necessary. A more general approach to supervised discriminative learning is based on the principle of **empirical risk minimization**. --- class: middle, center # Empirical risk minimization --- class: middle - Consider a function $f : \mathcal{X} \to \mathcal{Y}$ produced by some learning algorithm, which can depend on some parameters not represented here. $\hat{y} = f(\mathbf{x})$ is the prediction of the ground-truth label $y$ associated with $\mathbf{x}$. - The predictions of this function can be evaluated through a loss function $$\ell : \mathcal{Y} \times \mathcal{Y} \to \mathbb{R},$$ such that $\ell(y, f(\mathbf{x})) \geq 0$ measures how close the prediction $f(\mathbf{x})$ is from $y$. For example: - Classification: $\ell(y,f(\mathbf{x})) = \mathbf{1}\_{y \cancel= f(\mathbf{x})}$ - Regression: $\ell(y,f(\mathbf{x})) = (y - f(\mathbf{x}))^2$ .credit[Credits: Gilles Louppe, [INFO8010 - Deep Learning](https://github.com/glouppe/info8010-deep-learning), ULiège.] --- class: middle Let $\mathcal{F}$ denote the hypothesis space, i.e. the set of all functions $f$ than can be produced by the chosen learning algorithm. We are looking for a function $f \in \mathcal{F}$ with a small **expected risk** (or generalization error) $$R(f) = \mathbb{E}\_{p^\star(\mathbf{x},y)}\left[ \ell(y, f(\mathbf{x})) \right] = \mathbb{E}\_{p^\star(\mathbf{x})}\left[ \mathbb{E}\_{p^\star(y| \mathbf{x})}\left[ \ell(y, f(\mathbf{x})) \right] \right].$$ This means that for a given data generating distribution $p^\star(\mathbf{x},y)$ and for a given hypothesis space $\mathcal{F}$, the optimal model is $$f^\star = \arg \min\_{f \in \mathcal{F}} R(f).$$ .credit[Credits: Gilles Louppe, [INFO8010 - Deep Learning](https://github.com/glouppe/info8010-deep-learning), ULiège.] --- class: middle Unfortunately, since $p^\star(\mathbf{x},y)$ is unknown, the expected risk cannot be evaluated and the optimal model cannot be determined. However, if we have i.i.d. training data $\mathcal{D} = \\\{(\mathbf{x}\_i, y\_i) \\\}\_{i=1}^N$, we can compute an estimate, the **empirical risk** (or training error) $$\hat{R}(f, \mathcal{D}) = \frac{1}{N} \sum\_{(\mathbf{x}\_i, y\_i) \in \mathcal{D}} \ell(y\_i, f(\mathbf{x}\_i)).$$ This estimate is **unbiased** and can be used for finding a good enough approximation of $f^\star$. This results into the **empirical risk minimization principle**: $$f^\star\_{\mathcal{D}} = \arg \min\_{f \in \mathcal{F}} \hat{R}(f, \mathcal{D})$$ .credit[Credits: Gilles Louppe, [INFO8010 - Deep Learning](https://github.com/glouppe/info8010-deep-learning), ULiège.] ??? What does unbiased mean? => The expected empirical risk estimate (over d) is the expected risk. --- class: middle Most supervised machine learning algorithms, including **neural networks**, implement empirical risk minimization. Under some regularity assumptions, empirical risk minimizers converge: $$\lim\_{N \to \infty} f^\star\_{\mathcal{D}} = f^\star$$ .credit[Credits: Gilles Louppe, [INFO8010 - Deep Learning](https://github.com/glouppe/info8010-deep-learning), ULiège.] ??? This is why tuning the parameters of the model to make it work on the training data is a reasonable thing to do. --- ## Regression example .center[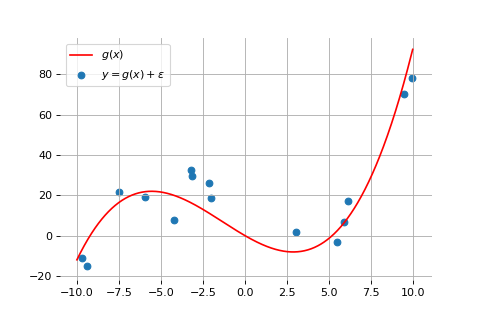] Consider the joint probability distribution $p^\star(x,y)$ induced by the data generating process $$(x,y) \sim p^\star(x,y) \Leftrightarrow x \sim \mathcal{U}([-10;10]), \epsilon \sim \mathcal{N}(0, \sigma^2), y = g(x) + \epsilon$$ where $x \in \mathbb{R}$, $y\in\mathbb{R}$ and $g$ is an unknown polynomial of degree 3. .credit[Credits: Gilles Louppe, [INFO8010 - Deep Learning](https://github.com/glouppe/info8010-deep-learning), ULiège.] --- .center.width-70[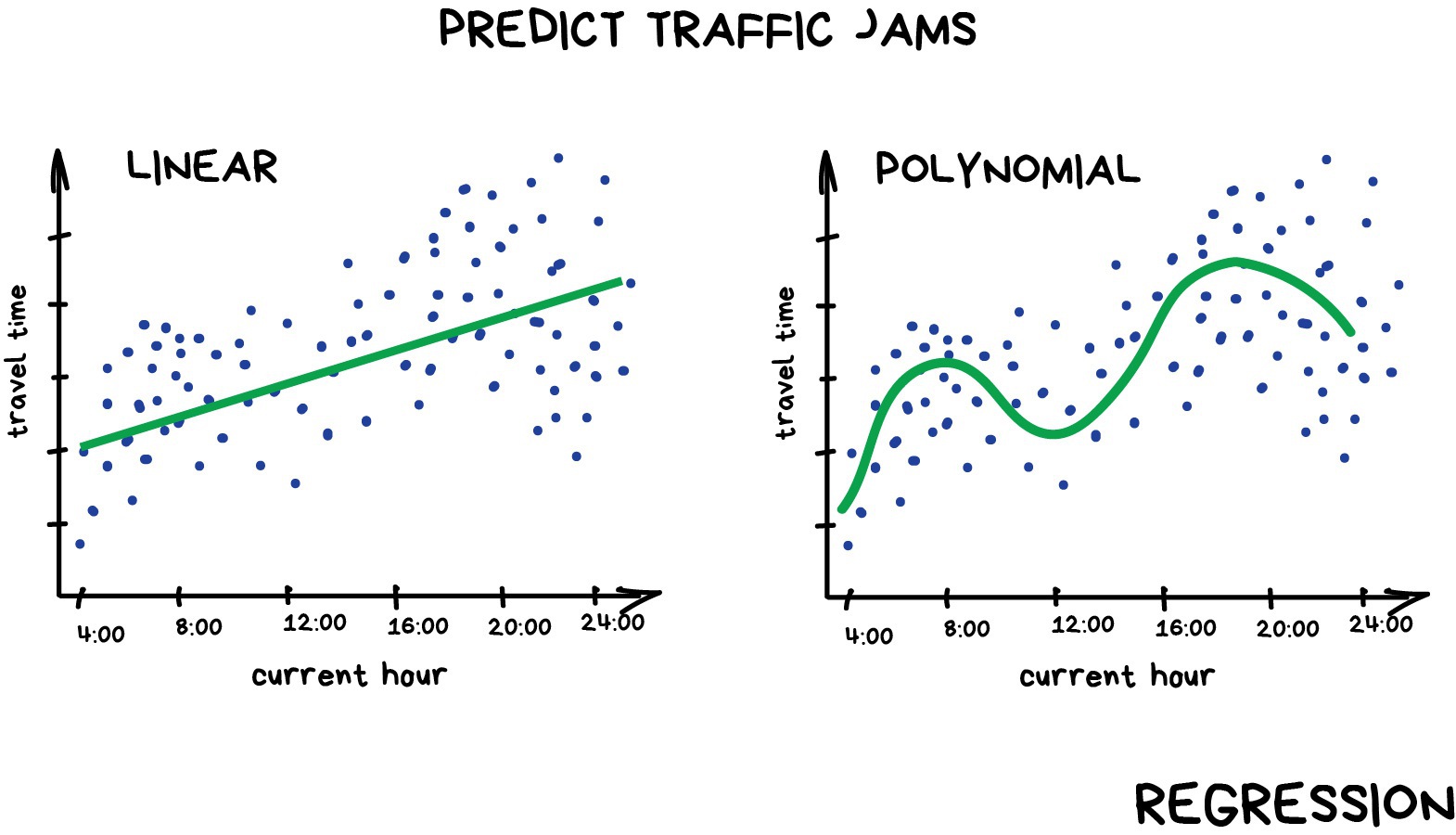] Regression is used to study the relationship between two continuous variables. Of course, it can be extended to higher dimensions. .credit[Image credit: https://noeliagorod.com/2019/05/21/machine-learning-for-everyone-in-simple-words-with-real-world-examples-yes-again/] ??? Regression examples: - predict the hospitalization time given your medical record - predict the rate for your insurance when taking a credit for buying a house given all your presonal data - predict your click rate in online advertising given your navigation history - predict the temperature given carbon emission rates - predict your age given a picture of you - predict a picture of you in 10 years given a picture of you today --- class: middle ## Step 1: Defining the model Our goal is to find a function $f$ that makes good predictions on average over $p^\star(x,y)$. Consider the hypothesis space $f \in \mathcal{F}$ of polynomials of degree 3 defined through their parameters $\mathbf{w} \in \mathbb{R}^4$ such that $$\hat{y} \triangleq f(x; \mathbf{w}) = \sum\_{d=0}^3 w\_d x^d$$ .credit[Credits: Gilles Louppe, [INFO8010 - Deep Learning](https://github.com/glouppe/info8010-deep-learning), ULiège.] --- class: middle ## Step 2: Defining the loss function For this regression problem, we use the squared error loss $$\ell(y, f(x;\mathbf{w})) = (y - f(x;\mathbf{w}))^2$$ to measure how wrong the predictions are. Therefore, our goal is to find the best value $\mathbf{w}^\star$ such $$\begin{aligned} \mathbf{w}^\star &= \arg\min\_\mathbf{w} R(\mathbf{w}) \\\\ &= \arg\min\_\mathbf{w} \mathbb{E}\_{p^\star(x,y)}\left[ (y-f(x;\mathbf{w}))^2 \right] \end{aligned}$$ .credit[Credits: Gilles Louppe, [INFO8010 - Deep Learning](https://github.com/glouppe/info8010-deep-learning), ULiège.] --- class: middle ## Step 3: Training Given a large enough training set $\mathcal{D} = \\\{(x\_i, y\_i) | i=1,\ldots,N\\\}$, the empirical risk minimization principle tells us that a good estimate $\mathbf{w}^\star\_{\mathcal{D}}$ of $\mathbf{w}^\star$ can be found by minimizing the empirical risk: $$\begin{aligned} \mathbf{w}^\star\_{\mathcal{D}} &= \arg\min\_\mathbf{w} \hat{R}(\mathbf{w},\mathcal{D}) \\\\ &= \arg\min\_\mathbf{w} \frac{1}{N} \sum\_{(x\_i, y\_i) \in \mathcal{D}} (y\_i - f(x\_i;\mathbf{w}))^2 \\\\ &= \arg\min\_\mathbf{w} \frac{1}{N} \sum\_{(x\_i, y\_i) \in \mathcal{D}} \Big(y\_i - \sum\_{d=0}^3 w\_d x\_i^d\Big)^2 \\\\ &= \arg\min\_\mathbf{w} \frac{1}{N} \left\lVert \underbrace{\begin{pmatrix} y\_1 \\\\ y\_2 \\\\ \ldots \\\\ y\_N \end{pmatrix}}\_{\mathbf{y}} - \underbrace{\begin{pmatrix} x\_1^0 \ldots x\_1^3 \\\\ x\_2^0 \ldots x\_2^3 \\\\ \ldots \\\\ x\_N^0 \ldots x\_N^3 \end{pmatrix}}\_{\mathbf{X}} \begin{pmatrix} w\_0 \\\\ w\_1 \\\\ w\_2 \\\\ w\_3 \end{pmatrix} \right\rVert^2 \end{aligned}$$ .credit[Credits: Gilles Louppe, [INFO8010 - Deep Learning](https://github.com/glouppe/info8010-deep-learning), ULiège.] --- class: middle This is **ordinary least squares** regression, for which the solution is known analytically: $$\mathbf{w}^\star\_{\mathcal{D}} = (\mathbf{X}^T\mathbf{X})^{-1}\mathbf{X}^T\mathbf{y}$$ .center[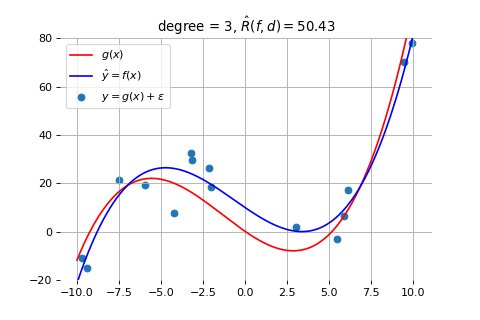] In many situations, the problem is more difficult and we cannot find the solution analytically. We resort to .bold[iterative optimization algorithms], such as (variants of) gradient descent. .credit[Credits: Gilles Louppe, [INFO8010 - Deep Learning](https://github.com/glouppe/info8010-deep-learning), ULiège.] --- class: middle The expected risk minimizer $f(x;\mathbf{w}^\star)$ within our hypothesis space $\mathcal{F}$ (polynomials of degree 3) is $g(x)$ itself (i.e. the polynomial of degree 3 with the true parameters). Therefore, on this toy problem, we can verify that $f(x;\mathbf{w}^\star\_{\mathcal{D}}) \to f(x;\mathbf{w}^\star) = g(x)$ as $N \to \infty$. .credit[Credits: Gilles Louppe, [INFO8010 - Deep Learning](https://github.com/glouppe/info8010-deep-learning), ULiège.] --- class: middle .center[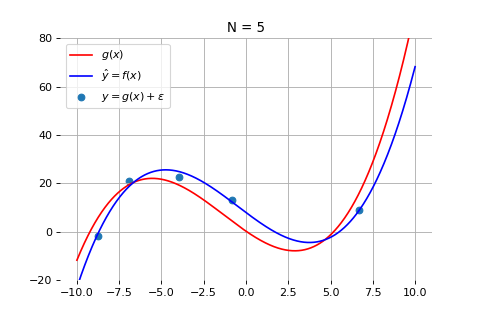] .credit[Credits: Gilles Louppe, [INFO8010 - Deep Learning](https://github.com/glouppe/info8010-deep-learning), ULiège.] --- class: middle count: false .center[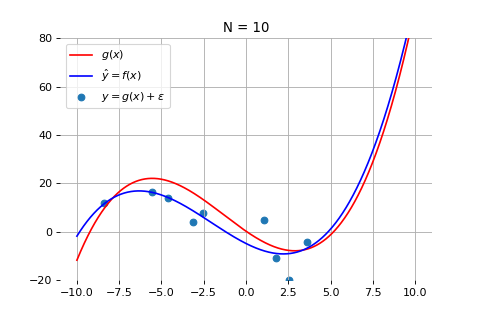] .credit[Credits: Gilles Louppe, [INFO8010 - Deep Learning](https://github.com/glouppe/info8010-deep-learning), ULiège.] --- class: middle count: false .center[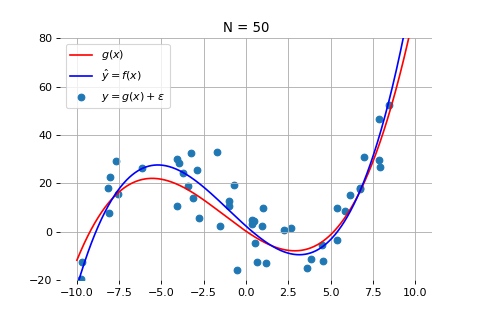] .credit[Credits: Gilles Louppe, [INFO8010 - Deep Learning](https://github.com/glouppe/info8010-deep-learning), ULiège.] --- class: middle count: false .center[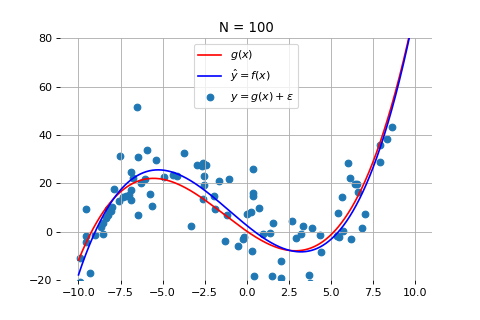] .credit[Credits: Gilles Louppe, [INFO8010 - Deep Learning](https://github.com/glouppe/info8010-deep-learning), ULiège.] --- class: middle count: false .center[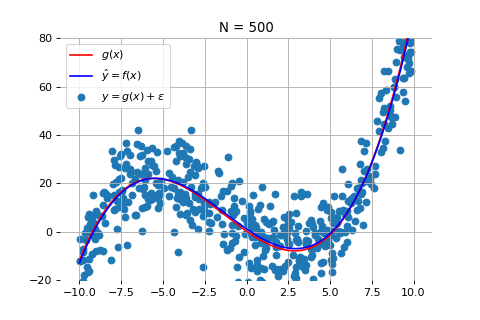] .credit[Credits: Gilles Louppe, [INFO8010 - Deep Learning](https://github.com/glouppe/info8010-deep-learning), ULiège.] --- class: middle What if we consider a hypothesis space $\mathcal{F}$ in which candidate functions $f$ are either too "simple" or too "complex" with respect to the true data generating process? .center[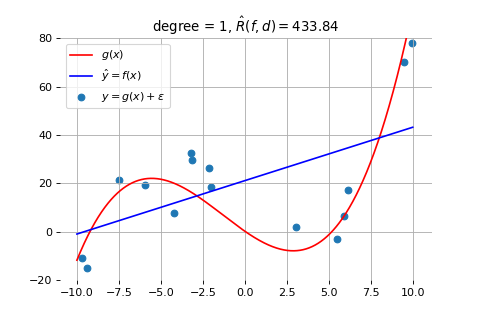] .center[$\mathcal{F}$ = polynomials of degree 1] .credit[Credits: Gilles Louppe, [INFO8010 - Deep Learning](https://github.com/glouppe/info8010-deep-learning), ULiège.] --- class: middle count: false What if we consider a hypothesis space $\mathcal{F}$ in which candidate functions $f$ are either too "simple" or too "complex" with respect to the true data generating process? .center[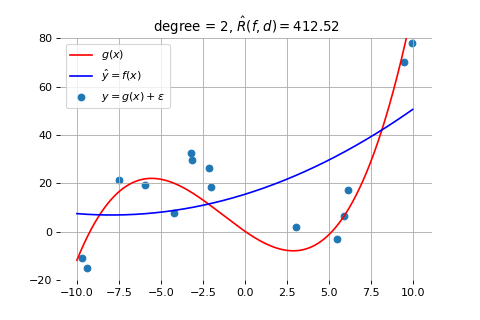] .center[$\mathcal{F}$ = polynomials of degree 2] .credit[Credits: Gilles Louppe, [INFO8010 - Deep Learning](https://github.com/glouppe/info8010-deep-learning), ULiège.] --- class: middle count: false What if we consider a hypothesis space $\mathcal{F}$ in which candidate functions $f$ are either too "simple" or too "complex" with respect to the true data generating process? .center[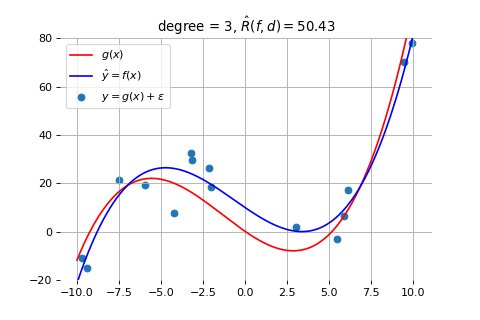] .center[$\mathcal{F}$ = polynomials of degree 3] .credit[Credits: Gilles Louppe, [INFO8010 - Deep Learning](https://github.com/glouppe/info8010-deep-learning), ULiège.] --- class: middle count: false What if we consider a hypothesis space $\mathcal{F}$ in which candidate functions $f$ are either too "simple" or too "complex" with respect to the true data generating process? .center[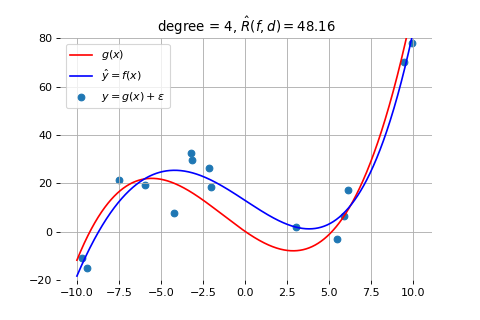] .center[$\mathcal{F}$ = polynomials of degree 4] .credit[Credits: Gilles Louppe, [INFO8010 - Deep Learning](https://github.com/glouppe/info8010-deep-learning), ULiège.] --- class: middle count: false What if we consider a hypothesis space $\mathcal{F}$ in which candidate functions $f$ are either too "simple" or too "complex" with respect to the true data generating process? .center[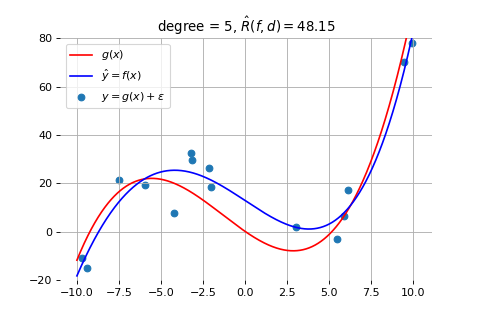] .center[$\mathcal{F}$ = polynomials of degree 5] .credit[Credits: Gilles Louppe, [INFO8010 - Deep Learning](https://github.com/glouppe/info8010-deep-learning), ULiège.] --- class: middle count: false What if we consider a hypothesis space $\mathcal{F}$ in which candidate functions $f$ are either too "simple" or too "complex" with respect to the true data generating process? .center[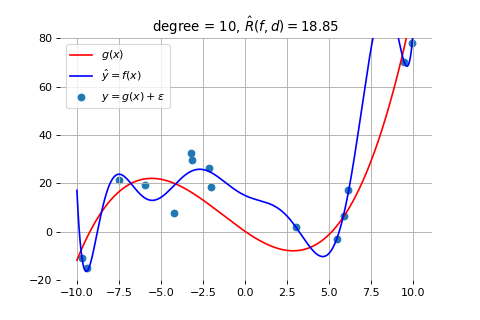] .center[$\mathcal{F}$ = polynomials of degree 10] .credit[Credits: Gilles Louppe, [INFO8010 - Deep Learning](https://github.com/glouppe/info8010-deep-learning), ULiège.] --- class: middle .center[ 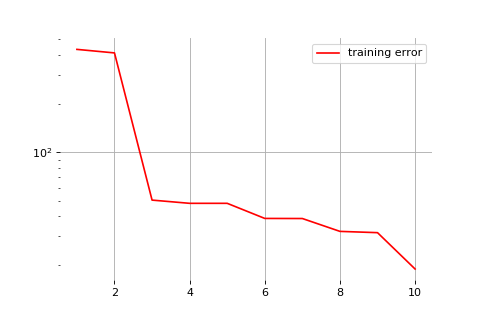 Error vs. degree $d$ of the polynomial. ] .credit[Credits: Gilles Louppe, [INFO8010 - Deep Learning](https://github.com/glouppe/info8010-deep-learning), ULiège.] ??? Why shouldn't we pick the largest $d$? --- class: middle ## Bayes risk and estimator Let $\mathcal{Y}^{\mathcal X}$ be the set of all functions $f : \mathcal{X} \to \mathcal{Y}$. We define the **Bayes risk** as the minimal expected risk over all possible functions, $$R\_B = \min\_{f \in \mathcal{Y}^{\mathcal X}} R(f),$$ and call **Bayes estimator** the model $f_B$ that achieves this minimum. .bold[No model $f$ can perform better than $f\_B$.] .credit[Credits: Gilles Louppe, [INFO8010 - Deep Learning](https://github.com/glouppe/info8010-deep-learning), ULiège.] --- class: middle The **capacity** of an hypothesis space $\mathcal{F}$ induced by a learning algorithm intuitively represents the ability to find a good model $f \in \mathcal{F}$ that can fit any function, regardless of its complexity. If the capacity is infinite, we can fit any function, but in practice the capacity is always finite. The capacity can be controlled through hyper-parameters of the learning algorithm. For example: - The degree of the family of polynomials; - The number of layers in a neural network; - The number of training iterations; - Regularization terms. .credit[Credits: Gilles Louppe, [INFO8010 - Deep Learning](https://github.com/glouppe/info8010-deep-learning), ULiège.] --- class: middle ## Underfitting and overfitting - If the capacity of $\mathcal{F}$ is too low, then $f\_B \notin \mathcal{F}$ and $R(f) - R\_B$ is large for any $f \in \mathcal{F}$, including $f^\star$ and $f^\star\_{\mathcal{D}}$. Such models $f$ are said to **underfit** the data. - If the capacity of $\mathcal{F}$ is too high, then $f\_B \in \mathcal{F}$ or $R(f^\star) - R\_B$ is small.
However, because of the high capacity of the hypothesis space, the empirical risk minimizer $f^\star\_{\mathcal{D}}$ could fit the training data arbitrarily well such that $$R(f^\star\_{\mathcal{D}}) \geq R\_B \geq \hat{R}(f^\star\_{\mathcal{D}}, \mathcal{D}) \geq 0.$$ This indicates that the empirical risk $\hat{R}(f^\star\_{\mathcal{D}}, \mathcal{D})$ is a poor estimator of the expected risk $R(f^\star\_{\mathcal{D}})$. In this situation, $f^\star\_{\mathcal{D}}$ becomes too specialized with respect to the true data generating process, $f^\star\_{\mathcal{D}}$ is said to **overfit** the data. .credit[Credits: Gilles Louppe, [INFO8010 - Deep Learning](https://github.com/glouppe/info8010-deep-learning), ULiège.] --- class: middle Therefore, our goal is to adjust the capacity of the hypothesis space such that the **expected risk** of the empirical risk minimizer (the generalization error) $R(f^\star\_{\mathcal{D}})$ gets as low as possible, and not simply the **empirical risk** of the empirical risk minimizer (training error) $\hat{R}(f^\star\_{\mathcal{D}}, \mathcal{D})$. .center[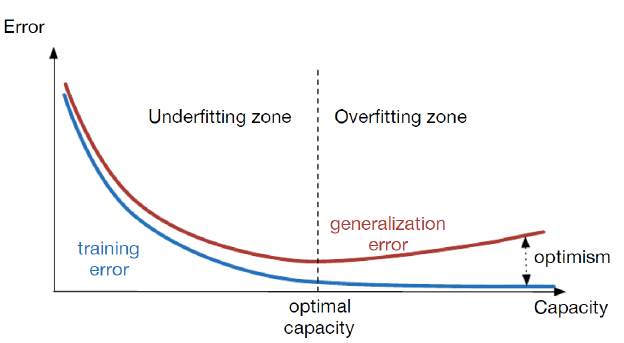] .credit[Credits: Gilles Louppe, [INFO8010 - Deep Learning](https://github.com/glouppe/info8010-deep-learning), ULiège.] ??? Comment that for deep networks, training error may goes to 0 while the generalization error may not necessarily go up! --- class: middle An unbiased estimate of the expected risk can be obtained by evaluating $f^\star\_{\mathcal{D}}$ on data $\mathcal{D}\_\text{test}$ independent from the training samples $\mathcal{D}$: $$\hat{R}(f^\star\_{\mathcal{D}}, \mathcal{D}\_\text{test}) = \frac{1}{N\_\text{test}} \sum\_{(\mathbf{x}\_i, y\_i) \in \mathcal{D}\_\text{test}} \ell(y\_i, f^\star\_{\mathcal{D}}(\mathbf{x}\_i))$$ This **test error** estimate can be used to evaluate the actual performance of the model. However, it should not be used, at the same time, for model selection. .credit[Credits: Gilles Louppe, [INFO8010 - Deep Learning](https://github.com/glouppe/info8010-deep-learning), ULiège.] --- class: middle .center[ 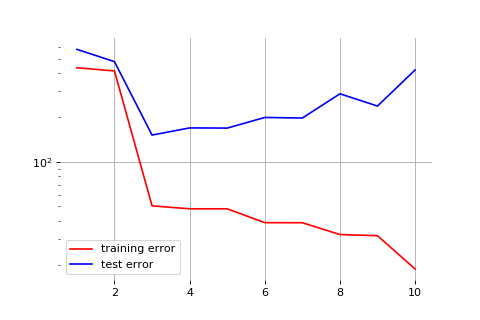 Error vs. degree $d$ of the polynomial. ] .credit[Credits: Gilles Louppe, [INFO8010 - Deep Learning](https://github.com/glouppe/info8010-deep-learning), ULiège.] ??? What value of $d$ shall you select? But then how good is this selected model? --- class: middle .center[] .center[This should be **avoided** at all costs!] .credit[Credits: Francois Fleuret, [EE559 Deep Learning](https://fleuret.org/ee559/), EPFL.] --- class: middle .center[] .center[Instead, keep a separate validation set for tuning the hyper-parameters.] .credit[Credits: Francois Fleuret, [EE559 Deep Learning](https://fleuret.org/ee559/), EPFL.] ??? Comment on the comparison of algorithms from one paper to the other. --- ## Bias-variance decomposition Consider a **fixed point** $\mathbf{x}$ and the prediction $\hat{y}=f^\star\_{\mathcal{D}}(\mathbf{x})$ of the empirical risk minimizer at $\mathbf{x}$. Then the local expected risk of $f^\star\_{\mathcal{D}}$ is $$\begin{aligned} R(f^\star\_{\mathcal{D}}|\mathbf{x}) &= \mathbb{E}\_{p^\star(y|\mathbf{x})} \left[ (y - f^\star\_{\mathcal{D}}(\mathbf{x}))^2 \right] \\\\ &= \mathbb{E}\_{p^\star(y|\mathbf{x})} \left[ (y - f\_B(\mathbf{x}) + f\_B(\mathbf{x}) - f^\star\_{\mathcal{D}}(\mathbf{x}))^2 \right] \\\\ &= \mathbb{E}\_{p^\star(y|\mathbf{x})} \left[ (y - f\_B(\mathbf{x}))^2 \right] + \mathbb{E}\_{ p^\star(y|\mathbf{x})} \left[ (f\_B(\mathbf{x}) - f^\star\_{\mathcal{D}}(\mathbf{x}))^2 \right] \\\\ &= R(f\_B|\mathbf{x}) + (f\_B(\mathbf{x}) - f^\star\_{\mathcal{D}}(\mathbf{x}))^2 \end{aligned}$$ - $R(f\_B|\mathbf{x})$ is the local expected risk of the Bayes estimator. This term cannot be reduced. - $(f\_B(\mathbf{x}) - f^\star\_{\mathcal{D}}(\mathbf{x}))^2$ represents the discrepancy between $f\_B$ and $f^\star\_{\mathcal{D}}$. .footnote[ Remarks: - $\scriptsize R(f) = \mathbb{E}\_{p^\star(\mathbf{\mathbf{x}},y)}\left[ \ell(y, f(\mathbf{\mathbf{x}})) \right] = \mathbb{E}\_{p^\star(\mathbf{\mathbf{x}})}\left[ \mathbb{E}\_{p^\star(y| \mathbf{\mathbf{x}})}\left[ \ell(y, f(\mathbf{\mathbf{x}})) \right] \right] = \mathbb{E}\_{p^\star(\mathbf{\mathbf{x}})}\left[ R(f | \mathbf{x}) \right]$ - To go from the second to third line we used the fact that $f\_B(\mathbf{x}) = \arg\min\_{f \in \mathcal{Y}^{\mathcal X}} R(f) = \mathbb{E}\_{p^\star(y|\mathbf{x})}[y]$. ] .credit[Credits: Gilles Louppe, [INFO8010 - Deep Learning](https://github.com/glouppe/info8010-deep-learning), ULiège.] --- class: middle If $\mathcal{D}$ is itself considered as a random variable, then $f^\star\_{\mathcal{D}}$ is also a random variable, along with its predictions $\hat{y} = f^\star\_{\mathcal{D}}(\mathbf{x})$. .credit[Credits: Gilles Louppe, [INFO8010 - Deep Learning](https://github.com/glouppe/info8010-deep-learning), ULiège.] --- class: middle count: false .center[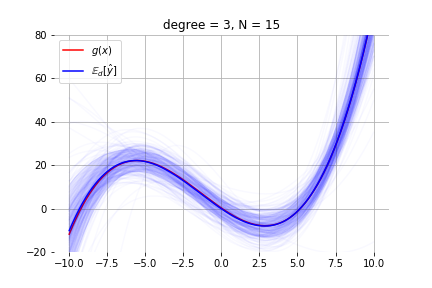] .credit[Credits: Gilles Louppe, [INFO8010 - Deep Learning](https://github.com/glouppe/info8010-deep-learning), ULiège.] --- class: middle Formally, the expected local expected risk yields to: $$\begin{aligned} &\mathbb{E}\_\mathcal{D} \left[ R(f^\star\_{\mathcal{D}}|\mathbf{x}) \right] \\\\ &= \mathbb{E}\_\mathcal{D} \left[ R(f\_B|\mathbf{x}) + (f\_B(\mathbf{x}) - f^\star\_{\mathcal{D}}(\mathbf{x}))^2 \right] \\\\ &= R(f\_B|\mathbf{x}) + \mathbb{E}\_\mathcal{D} \left[ (f\_B(\mathbf{x}) - f^\star\_{\mathcal{D}}(\mathbf{x}))^2 \right] \\\\ &= \underbrace{R(f\_B|\mathbf{x})}\_{\text{noise}} + \underbrace{(f\_B(\mathbf{x}) - \mathbb{E}\_\mathcal{D}\left[ f^\star\_{\mathcal{D}}(\mathbf{x}) \right] )^2}\_{\text{bias}^2} + \underbrace{\mathbb{E}\_\mathcal{D}\left[ ( f^\star\_{\mathcal{D}}(\mathbf{x}) - \mathbb{E}\_\mathcal{D}\left[ f^\star\_{\mathcal{D}}(\mathbf{x}) \right])^2 \right]}\_{\text{var}} \end{aligned}$$ This decomposition is known as the **bias-variance** decomposition. - The noise term quantity is the irreducible part of the expected risk. - The bias term measures the discrepancy between the average model and the Bayes estimator. - The variance term quantities the variability of the predictions. .credit[Credits: Gilles Louppe, [INFO8010 - Deep Learning](https://github.com/glouppe/info8010-deep-learning), ULiège.] --- class: middle ## Bias-variance trade-off - Reducing the capacity makes $f^\star\_{\mathcal{D}}$ fit the data less on average, which increases the bias term. - Increasing the capacity makes $f^\star\_{\mathcal{D}}$ vary a lot with the training data, which increases the variance term. .credit[Credits: Francois Fleuret, [EE559 Deep Learning](https://fleuret.org/ee559/), EPFL.] --- class: middle .center[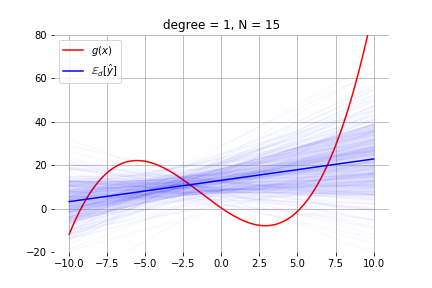] .credit[Credits: Gilles Louppe, [INFO8010 - Deep Learning](https://github.com/glouppe/info8010-deep-learning), ULiège.] ??? What do you observe? --- class: middle count: false .center[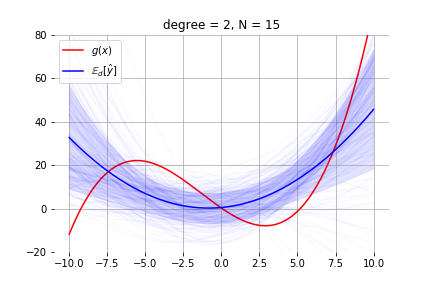] .credit[Credits: Gilles Louppe, [INFO8010 - Deep Learning](https://github.com/glouppe/info8010-deep-learning), ULiège.] --- class: middle count: false .center[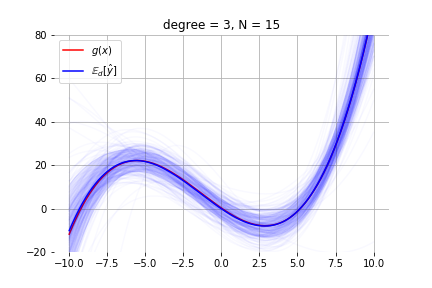] .credit[Credits: Gilles Louppe, [INFO8010 - Deep Learning](https://github.com/glouppe/info8010-deep-learning), ULiège.] --- class: middle count: false .center[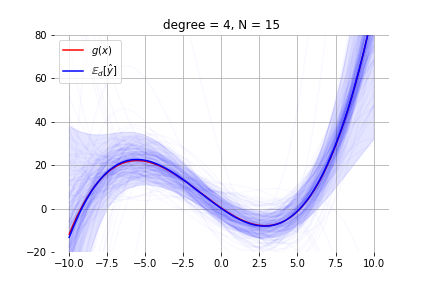] .credit[Credits: Gilles Louppe, [INFO8010 - Deep Learning](https://github.com/glouppe/info8010-deep-learning), ULiège.] --- class: middle count: false .center[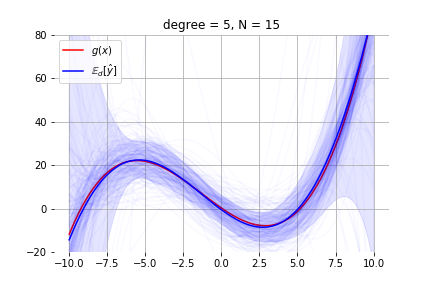] .credit[Credits: Gilles Louppe, [INFO8010 - Deep Learning](https://github.com/glouppe/info8010-deep-learning), ULiège.] --- class: middle, center ## Lab session on multinomial logistic regression .center.width-30[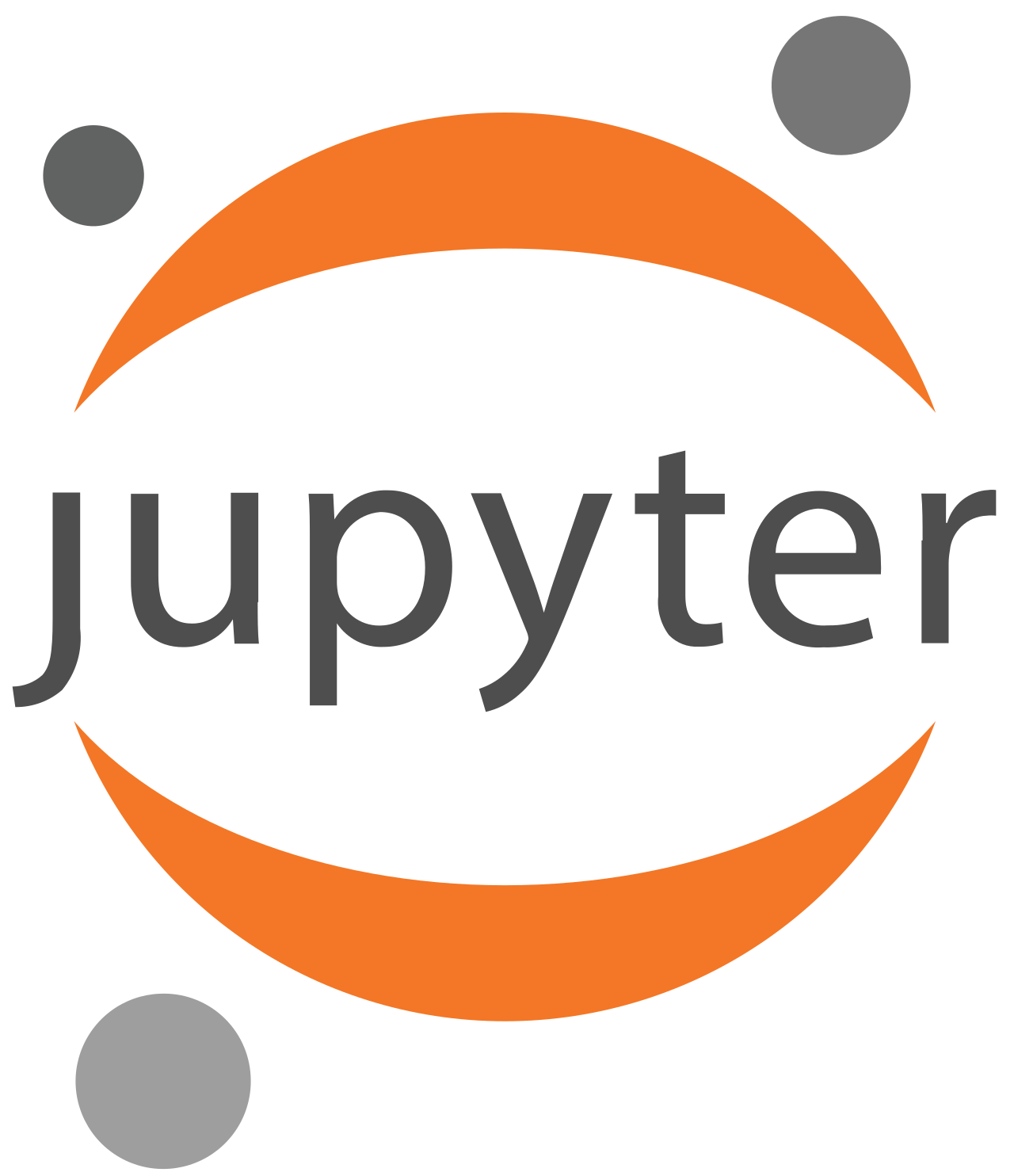] --- class: middle, center # Appendix: 3 fundamental examples --- class: middle ## Binary classification example - **Training data**: $(\mathbf{x}, y) \in \mathcal{X} \times \mathcal{Y}$ with $\mathcal{X} = \mathbb{R}^D$ and $ \mathcal{Y} = \\\{0, 1\\\}$. - **Model**: We assume there exists a function $f(\cdot; \theta): \mathbb{R}^D \mapsto [0, 1]$ such that $p(y=1|\mathbf{x} ; \theta) = f(\mathbf{x}; \theta)$ and $p(y=0|\mathbf{x} ; \theta) = 1 - f(\mathbf{x}; \theta)$. The model can be compactly rewritten using the following probability mass function (pmf) for all $y \in \mathcal{Y}$: $$ p(y \mid \mathbf{x} ; \theta) = \Big(f(\mathbf{x}; \theta)\Big)^y \Big(1-f(\mathbf{x}; \theta)\Big)^{(1-y)}. $$ - The **NLL** function is called the **binary cross-entropy**: $$- \ln p(y \mid \mathbf{x} ; \theta) = - y \ln\Big(f(\mathbf{x}; \theta)\Big) - (1-y) \ln\Big(1-f(\mathbf{x}; \theta)\Big). $$ --- class: middle ## $C$-class classification example - **Training data**: $(\mathbf{x}, y) \in \mathcal{X} \times \mathcal{Y}$ with $\mathcal{X} = \mathbb{R}^D$ and $ \mathcal{Y} = \\\{1,...,C\\\}$. - **Model**: We assume there exists a function $f(\cdot; \theta): \mathbb{R}^D \mapsto [0, 1]^C$ such that $p(y=k \mid \mathbf{x} ; \theta) = f\_k(\mathbf{x}; \theta)$ for all $k \in \\\{1,...,C\\\}$ and $\sum\limits\_{k=1}^C f_k(\mathbf{x}; \theta) = 1$. It can be compactly rewritten using the following pmf for all $y \in \mathcal{Y}$: $$ p(y \mid \mathbf{x} ; \theta) = \prod\_{k=1}^{C} p(y=k \mid \mathbf{x} ; \theta)^{\mathbf{1}\_{y = k}} = \prod\_{k=1}^{C} f\_k(\mathbf{x}; \theta)^{\mathbf{1}\_{y = k}} . $$ - The **NLL** function is called the **cross-entropy**: $$- \ln p(y \mid \mathbf{x} ; \theta) = - \sum\_{k=1}^{C} \mathbf{1}\_{y = k} \ln\Big( f\_k(\mathbf{x}; \theta) \Big).$$ --- class: middle ## Logistic regression vs. naive Bayes classifiers - When $f(\mathbf{x}; \theta)$ is an affine function of $\mathbf{x}$, this model is called (multinomial) [logistic regression](https://en.wikipedia.org/wiki/Multinomial_logistic_regression). It is one of the simplest discriminative models for supervised classification. - The most popular generative model for supervised classification is called the [naive Bayes](https://en.wikipedia.org/wiki/Naive_Bayes_classifier) classifier. - For a comparison between the generative and discriminative approches to supervised classification, see [(Ng and Jordan, 2002)](http://papers.nips.cc/paper/2020-on-discriminative-vs-generative-classifiers-a-comparison-of-logistic-regression-and-naive-bayes). .credit[Ng, Andrew Y.; Jordan, Michael I. (2002). [On discriminative vs. generative classifiers: A comparison of logistic regression and naive Bayes](http://papers.nips.cc/paper/2020-on-discriminative-vs-generative-classifiers-a-comparison-of-logistic-regression-and-naive-bayes). Advances in Neural Information Processing Systems (NIPS).] --- class: middle ## Regression example - **Training data**: $(\mathbf{x}, \mathbf{y}) \in \mathcal{X} \times \mathcal{Y}$ with $\mathcal{X} = \mathbb{R}^P$ and $ \mathcal{Y} = \mathbb{R}^Q$. - **Model**: We assume there exists a function $f(\cdot; \theta): \mathbb{R}^P \mapsto \mathbb{R}^Q$ such that $p(\mathbf{y} \mid \mathbf{x}; \theta) = \mathcal{N}\Big(\mathbf{y}; f(\mathbf{x}; \theta), \mathbf{I} \Big) = (2 \pi)^{-Q/2} \exp\Big( - \frac{1}{2} \parallel \mathbf{y} - f(\mathbf{x}; \theta) \parallel_2^2 \Big)$. - The **NLL** gives the **squared error**: $$- \ln p(\mathbf{y} \mid \mathbf{x} ; \theta) = \frac{1}{2} \parallel \mathbf{y} - f(\mathbf{x}; \theta) \parallel_2^2 + \,cst.$$